AI Text Detection: Understanding Machine Learning Content Analysis
In the digital age, the proliferation of content across various platforms has necessitated the development of sophisticated tools to analyze and understand this vast amount of information. Machine learning content analysis has emerged as a pivotal technology in this realm, offering powerful solutions for processing and interpreting textual data. This field leverages algorithms and statistical models to extract meaningful insights from text, enabling applications such as sentiment analysis, topic detection, and language translation.
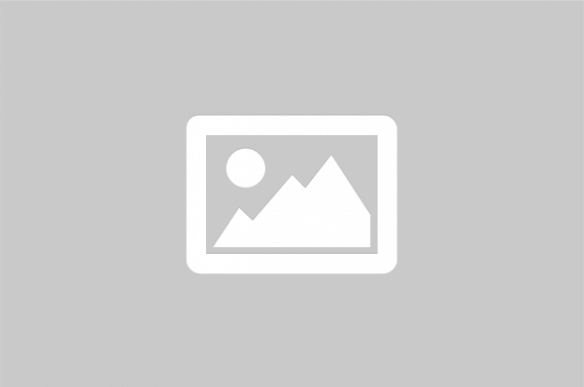
Machine learning content analysis is not just about understanding text; it involves a comprehensive approach to processing natural language, recognizing patterns, and making predictions. This technology is integral to various sectors, including marketing, customer service, and media, where it aids in personalizing user experiences and enhancing engagement. The ability to analyze sentiment, for instance, allows companies to gauge public opinion and adjust their strategies accordingly. Moreover, machine learning content analysis facilitates the categorization of vast amounts of data, making it easier to manage and retrieve information. As we delve deeper into this topic, we will explore the methodologies, applications, and future prospects of machine learning content analysis, highlighting its transformative impact on the digital landscape.
Machine learning content analysis is a transformative technology that enables the automated understanding and processing of text data. It employs various algorithms to analyze and interpret textual information, providing insights that are crucial for decision-making across different sectors. This technology is particularly valuable in handling unstructured data, which is abundant in today’s digital world. By converting unstructured text into structured data, machine learning facilitates better data management and retrieval, enhancing the overall efficiency of information processing.
Key Techniques in Machine Learning Content Analysis
Several techniques are employed in machine learning content analysis to extract meaningful insights from text:
- Natural Language Processing (NLP): NLP is a branch of artificial intelligence that focuses on the interaction between computers and humans through natural language. It enables machines to understand and interpret human language, making it a cornerstone of content analysis.
- Sentiment Analysis: This technique involves determining the sentiment or emotion expressed in a piece of text. It is widely used in social media monitoring and customer feedback analysis to gauge public opinion and sentiment.
- Topic Modeling: Topic modeling is used to identify the underlying themes or topics present in a large corpus of text. It helps in organizing and categorizing information, making it easier to analyze and understand.
- Text Classification: This involves categorizing text into predefined classes or categories. It is used in applications such as spam detection, news categorization, and sentiment analysis.
Applications of Machine Learning Content Analysis
Machine learning content analysis has a wide range of applications across various industries:
- Marketing: By analyzing customer feedback and social media interactions, companies can tailor their marketing strategies to better meet customer needs and preferences.
- Customer Service: Automated chatbots and virtual assistants use content analysis to understand and respond to customer queries effectively, improving the overall customer experience.
- Media and Journalism: Content analysis helps in identifying trending topics and public sentiment, enabling media outlets to deliver relevant and timely news.
- Healthcare: In the healthcare sector, content analysis is used to process and analyze medical records, research papers, and patient feedback, aiding in better decision-making and patient care.
Comparison of Machine Learning Content Analysis Tools
Tool | Features | Applications | Price |
---|---|---|---|
Google Cloud Natural Language API | Sentiment analysis, entity recognition, syntax analysis | Customer feedback analysis, content categorization | Pay-as-you-go |
IBM Watson Natural Language Understanding | Emotion analysis, keyword extraction, semantic roles | Market research, media monitoring | Custom pricing |
Amazon Comprehend | Language detection, key phrase extraction, sentiment analysis | Social media monitoring, document classification | Pay-as-you-go |
Microsoft Azure Text Analytics | Sentiment analysis, entity recognition, language detection | Business intelligence, customer insights | Custom pricing |
Future Prospects of Machine Learning Content Analysis
The future of machine learning content analysis is promising, with advancements in technology paving the way for more sophisticated and accurate analysis. As algorithms become more refined, the ability to understand and interpret complex human language will improve, leading to more personalized and effective applications. Additionally, the integration of machine learning with other technologies such as big data and the Internet of Things (IoT) will expand the capabilities of content analysis, enabling real-time insights and decision-making.
Furthermore, ethical considerations and data privacy will play a significant role in shaping the future of content analysis. Ensuring that data is used responsibly and ethically will be crucial in maintaining public trust and compliance with regulations. As the field continues to evolve, collaboration between technologists, policymakers, and industry leaders will be essential in harnessing the full potential of machine learning content analysis.
References: Google Cloud Natural Language API , IBM Watson Natural Language Understanding , Amazon Comprehend , Microsoft Azure Text Analytics